Easily Identify your Best Customers
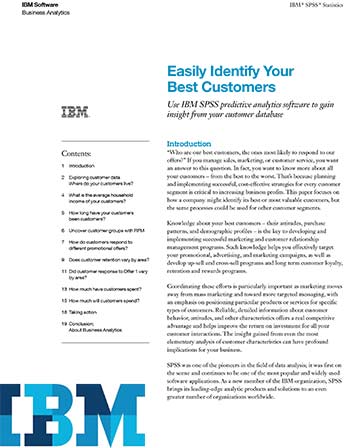
Knowledge about your best customers – their attitudes, purchase patterns, and demographic profiles – is the key to developing and implementing successful marketing and customer relationship management programs. Such knowledge helps you effectively target your promotional, advertising, and marketing campaigns, as well as develop up-sell and cross-sell programs and long term customer loyalty, retention and rewards programs. This white paper outlines how predictive analytics can help you gain valuable insight from your customer database.
Get the downloadBelow is an excerpt of "Easily Identify your Best Customers". To get your free download, and unlimited access to the whole of bizibl.com, simply log in or join free. |
![]() |
Introduction
“Who are our best customers, the ones most likely to respond to our offers?” If you manage sales, marketing, or customer service, you want an answer to this question. In fact, you want to know more about all your customers – from the best to the worst. That’s because planning and implementing successful, cost-effective strategies for every customer segment is critical to increasing business profits. This paper focuses on how a company might identify its best or most valuable customers, but the same processes could be used for other customer segments.
Knowledge about your best customers – their attitudes, purchase patterns, and demographic profiles – is the key to developing and implementing successful marketing and customer relationship management programs. Such knowledge helps you effectively target your promotional, advertising, and marketing campaigns, as well as develop up-sell and cross-sell programs and long term customer loyalty, retention and rewards programs.
Coordinating these efforts is particularly important as marketing moves away from mass marketing and toward more targeted messaging, with an emphasis on positioning particular products or services for specific types of customers. Reliable, detailed information about customer behavior, attitudes, and other characteristics offers a real competitive advantage and helps improve the return on investment for all your customer interactions. The insight gained from even the most elementary analysis of customer characteristics can have profound implications for your business.
SPSS was one of the pioneers in the field of data analysis; it was first on the scene and continues to be one of the most popular and widely used software applications. As a new member of the IBM organization, SPSS brings its leading-edge analytic products and solutions to an even greater number of organizations worldwide.
IBM SPSS offerings include industry-leading products for data and text mining, data collection, management, and statistics software for identifying your best customers and planning more efficient and cost-effective marketing programs.
IBM SPSS tools are based on industry standards and can easily integrate with your existing infrastructure to improve accuracy, decrease manpower and minimize loss. The combined effort of IBM and SPSS brings you the utmost in flexibility in the kinds of data you mine and how you deploy results.
This white paper demonstrates how you could analyze a customer database using IBM SPSS predictive analytics software. This integrated product family for statistical analysis and data management supports you throughout the analytic process, whether you perform your analysis from a single desktop computer or across an extended network.
The marketing data we will use for the examples in this paper contains 2,070 customers and includes the following information:
- Date when customer first became a customer
- Purchase history by dollar value of orders
- Response to different offers
- Customer churn or turnover information
- Household income level
- Geographic classification
- Gender and other demographic variables
Our goal is to identify unique customer segments or groups which make up our company’s best customers. We’ll also explain how you can use IBM SPSS software to gain insight from your customer data, predict your customers’ future behavior, and make better business decisions.
Exploring customer data
We begin by exploring the different variables in our database to answer questions such as:
- Where do your customers live?
- What is the average household income?
- How long have your customers been customers?
- How much money do your customers spend with us?
IBM SPSS software offers several methods to quickly obtain the answers to these questions. The Frequencies and Descriptive procedures in IBM® SPSS® Statistics are very useful when taking a first look at your data. Often, this exercise can suggest the best methods for analyzing your data.
Where do your customers live?
Identifying where your customers live – whether they live in an urban, suburban or rural area – can help you determine the best marketing strategy for reaching them. The Frequencies procedure in IBM SPSS Statistics provides a table of counts and percents by category, along with a visual representation of the data in a bar, histogram or pie chart, complete with category labels assigned to each value.
From the results shown in Chart 1, we learn that the largest proportion of the customer base lives in a suburban area (34.2%), and the smallest proportion lives in a rural area (19.4%). We also see that 16.9 percent is listed as missing data, which are cases from whom no residential information was supplied.
[Download PDF to see Chart 1]
It is often useful to know where and why information is missing in your data. IBM SPSS Statistics recognizes missing data as either a complete null, or as a residual value like “not applicable” or “no opinion.” This enables analysts to distinguish between data that is missing because the question or observation does not apply to the respondent, and data that is missing because no response was provided.
Table 1 on the following page displays the results from a frequency distribution. The values displayed in the column labeled “Percent” are calculated using all the cases in the data set (N = 2070). The “Valid percent” column displays the proportionate distribution of cases with only valid data reported, or non-missing data (N = 1720). This provides a fast side-by-side comparison of the distributions; large differences between these two columns may suggest bias in the data. Table 1 also shows that nearly 17% of the data is missing, which could indicate potential issues for the analyses.
[Download PDF to see Table 1]
What is the average household income of your customers?
There are several ways to gain a more detailed view of your customers. To obtain information about household income, for example, we employ basic summary statistics, such as the mean, minimum and maximum values, and the standard deviation. The Descriptive procedure in IBM SPSS Statistics provides an informative first glimpse into your scale or interval level data, like income (measured in dollars). Table 2 below displays the descriptive summaries for household income.
[Download PDF to see Table 2]
We see in Table 2 that the average annual household income of the nearly 2,000 customers who reported their income in our data is $61,386.39. Looking at the standard deviation of approximately $11,000, we know that the majority of customers (approximately 68%) earn between $50,000 and $72,000.
How long have your customers been customers?
To determine how long your customers remain with you, derive a new field in the data using the date when the customer was first entered into the database. Subtracting that from the current date, or the date of their most recent transaction, you can then determine how long a customer has been your customer. By using one of the many time functions available in IBM SPSS Statistics, you can easily transform the date into the number of years since you acquired the customer.
Also in the database is churn information, or the status of the customer as current or having defected. Using this information and the length of time the customer has been in the database, you can determine customer “survival” or that amount of time a customer remains loyal before defecting.
Kaplan-Meyer Survival Analysis is a particularly useful way to measure the time until a customer becomes inactive or is no longer a customer. An important advantage of Kaplan-Meyer Survival Analysis is that this method accounts for customer loss from the database before churn can be determined: for example, if a customer remains as “current” but has been inactive. From Chart 2, below, we can see that as length of time in the database increases, fewer customers remain as active. In other words, the cumulative proportion of customers remaining in the database steadily decreases as time increases. We can see that the median survival time – or that time at which 50 percent of the customers have churned – is approximately 11 years.
[Download PDF to see Chart 2]
Uncover customer groups with recency, frequency, and monetary value (RFM) analysis
Next, you can determine who your best customers are. “Best customers” are typically defined as the most profitable customers or the ones that spend the most money with your organization. To obtain the most accurate picture of customer lifetime value, we rely on recency, frequency and monetary value (RFM) analysis. For example, we can classify customers according to:
- Those who have spent the most – the most often and most recently.
- Those who have spent the most – the most monetarily, but may not have purchased in a long time.
- Those who spend the most in the fewest number of transactions.
- Those who spend the least, or rarely, and have not purchased in a long time.
With this capability, you can determine which of your customers are the best customers based on the recency of purchase, frequency of purchase and the amount that the customer spent.
Using RFM analysis within IBM SPSS Statistics enables you to generate a list of those customers who have spent the most by plugging in the appropriate variables, the customer ID, the transaction date and the transaction amount. In this instance, it is necessary to have a customer ID associated with every transaction. Transaction date is important so that you know when or how often the customer buys. Finally, because you will want to know how much a customer has purchased within their lifetime, you will also include the total.
[Download PDF to see Figure 1]
Once you have input these variables, you can run the analysis to get your customers’ RFM scores and determine which customers you want to target. In this instance, you might focus on those customers who have an RFM score of 555, which means they buy the most recently and frequently and spend the most money. Once the output is produced, you can then sort the data to focus on your top customers with an RFM score of 555.
[Download PDF to see Figure 2]
By using other descriptive analyses of customer spending, you can see that the majority of customers spent $500 or less and that at higher dollar-value levels the number of customers making purchases steadily declines. The average amount spent by customers is $1,360, and a very small number of customers spent in excess of $7,000.
So far, we know that a typical customer:
- Lives in a suburban area
- Has a household income of $61,000
- Spends $1,360 on our products and services
- Has a median “life span” or survival of 11 years
How do customers respond to different promotional offers?
IBM SPSS Statistics’ results analysis of specific marketing promotions is an important step toward understanding your customers. Evaluating past efforts helps identify what worked and what did not, so you can duplicate your successes and learn from your failures. Here, we want to answer two questions:
- How many people responded to each of our four offers?
- What is the average amount spent in response to our different promotions?
Want more like this?
Want more like this?
Insight delivered to your inbox
Keep up to date with our free email. Hand picked whitepapers and posts from our blog, as well as exclusive videos and webinar invitations keep our Users one step ahead.
By clicking 'SIGN UP', you agree to our Terms of Use and Privacy Policy
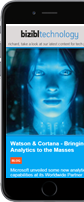
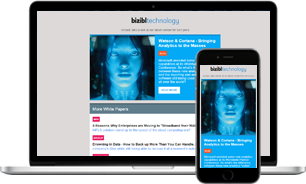
By clicking 'SIGN UP', you agree to our Terms of Use and Privacy Policy
To do so, we run the Frequencies procedure on each offer response and the Descriptive procedure on the order value for the four offers. on the order value for the four offers. In Table 4, we see that 890 customers, or 44.5 percent of the customer database, responded to Offer 1. Similar analysis of the other offers would show a 39 percent response to Offer 2, a 37.4 percent response to Offer 3 and a 17.4 percent response to Offer 4.
[Download PDF to see Figure 3]
From this we know that Offer 1 had the highest response rate, but not how those responses translated into revenue for the company. Running the Descriptive procedure on Offers 1 through 4 reveals that the value from Offer 1, $376.64, was also the best of the four offers, as shown in Table 4, while Offer 3, which also had a very high response rate, was the worst with $293.98 per response. So Offer 1 was, by both measures, more successful.
[Download PDF to see Table 4]
Does customer retention vary by area?
To explore this question, we generate a powerful statistical chart, the boxplot. This displays both the median value and the distribution of the data. From the boxplot in Chart 3, we can see that customers in rural areas have a greater median value for length of time in the database, which suggests that they have been customers longer, on average, than those in other areas.
[Download PDF to see Chart 3]
A Comparison of Means provides summary statistics for a measurement value by group. Table 5 complements the information displayed in the boxplot, but in a table format. It reveals that while the overall average length of time in the database is 7.49 years, customers in rural areas have remained customers longer than those in suburban or urban areas.
[Download PDF to see Table 5]
Is this a significant finding? Statistical significance tells us whether the differences we see in our data likely occurred by accident or not, or if those differences are likely to reflect patterns in the larger population and justify further attention.
The ANOVA report in Table 6 shows that the differences between area for length of time as customers are statistically significant. Traditionally, we consider something statistically significant when the probability of it occurring by accident is less than 5 percent, or fewer than 5 times in 100. This is indicated by a significance level of .05 or less. Since the level of significance reported in this table is .000, well under the .05 threshold, we can conclude that the difference in the means which we observe in the data likely did not occur by accident. The overall distribution of average customer retention and area is probably not due to random causes, but to something else.
[Download PDF to see Table 6]
Examples of possible causes include:
- The first office was opened in a rural area
- There is more need for the product in one area than in another
- A certain product feature was introduced successfully in one area
Other causes may exist and bear investigation. This is why it is also important to know your business, in order to gather the right data to test your theories about relationships.
Did customer response to Offer 1 vary by area?
Next, we continue our analysis of offer response. IBM SPSS Statistics provides an easy way to graphically present information on all four offers, using a clustered bar chart. Chart 4 provides a summary of response patterns by area. We see that customers in urban areas tend to under-order relative to the other two, particularly the rural. This is a finding we could not have guessed by looking at the frequency distribution of area, which showed us that the rural areas contained fewer people.
[Download PDF to see Chart 4]
To find out if this is significant, we can further explore the results of individual offers by area. To answer the question “How did people in each area respond to Offer 1?” we perform a IBM SPSS Statistics crosstab on Offer 1 by area. Table 7 shows 41.3 percent of the people who responded to Offer 1 were from suburban areas. While only 26.5 percent of the people who responded to Offer 1 were from rural areas, over half (50.5 percent) of the rural customers responded to the offer.
[Download PDF to see Table 7]
To understand whether area is associated with response to Offer 1, we compare the percentages in the “% of area” rows and find that 45 percent of people from suburban areas responded to this offer, and that 40 percent of people in urban areas responded. Based on this information, we conclude rural areas are good areas for an offer such as Offer 1.
[Download PDF to see Table 8]
However, while it appears the percentages are different, that is an insufficient reason to start duplicating Offer 1 in rural areas. First, we must determine whether area and response to Offer 1 are independent of each other. Here, the Chi-square statistic is useful in determining whether the distributions seen in the data are reflective of patterns in the larger population.
Table 9 contains Chi-square information for the area and Offer 1. In this case, the Chi-square is significant (p = .007) and indicates that the patterns in the table likely did not occur by accident. There could be a specific, identifiable reason that made Offer 1 more successful in rural areas. Perhaps the copy spoke more directly to their needs, or the media type was better matched to attract and keep their attention.
[Download PDF to see Table 9]
By identifying what made the campaign successful in rural areas, we can leverage that knowledge in future offers to this area. We also may choose to explore other relationships relative to area.
How much have customers spent?
Once again, using RFM analysis, we are able to plug in the variables to determine what we want to know. In this case, we want to know which customers have spent the most money. Once we run the analysis, we are then able to sort by dollar value for total transactions.
Another way to look at purchase history is to assess total amount spent, rather than just the money spent on individual orders. Perhaps a relationship between total money spent and area will reveal some insights. A one-way ANOVA provides specific information about the significance of the differences in average values that you may see.
The first thing that one-way ANOVA provides is a table of descriptive statistics. Table 9 shows that the average total amount spent in response to each of the four offers by area varies widely. In urban areas, the average amount spent was $1,206.01; in suburban areas, $1,391.70 was the average amount spent; while in rural areas, the average amount spent was $1,618.27.
The report also shows that the average difference exhibited between the spending levels in the suburban and the rural areas is not statistically significant. On the other hand, it shows that the difference between the rural and urban areas is significant.
You can use this information to further explore how and why these areas differ and develop targeted marketing plans to leverage the differences. For example, a different marketing and sales mix, different offer, or special bundle of products and services may work better in the urban areas. The marketing programs in rural areas should be repeated there for continued success.
How much will customers spend?
Predictive models are powerful tools to help target prospects and optimize marketing resources. They help answer questions such as “How much will customers spend, given their income level?”
In many statistical studies, the goal is to establish a relationship, expressed as an equation, for predicting typical values of one variable given the value of another. IBM SPSS Statistics offers several procedures for establishing relationships and defining predictive models. These procedures include scatterplots and correlations, linear and logistic regression analysis and classification trees. With the step-by-step instructions and help features built into the IBM SPSS Statistics product family, you can perform these procedures successfully, even if you aren’t a statistician.
Chart 5 shows the shape of the relationship between these two variables. The scatterplot is the correct chart to display the joint distribution of two continuous or interval variables. The correlation coefficient of .608, displayed in Table 10, indicates a strong and positive relationship between household income and total money spent. Regression analysis further defines the relationship with a model, as shown in Table 11. This relationship shows that as household income increases, the total money spent on products increases proportionately. This is valuable information which, if combined with more information about your customers, can be used to predict how much each customer is likely to spend.
[Download PDF to see Chart 5]
With IBM® SPSS® Decision Trees, we can identify unique segments within our database, based on each customer’s likelihood of having a specific characteristic or behavior that we are interested in predicting. This is illustrated in Chart 6, below:
[Download PDF to see Chart 6]
[Download PDF to see Tables 10 & 11]
To begin the analysis, we put information about area, product class category, and household income into a model in order to find out which customers are most likely to respond to Offer 1. IBM SPSS Decision Trees can use one of four established tree-growing algorithms to build a tree diagram of the results, as shown in Chart 6.
Income is found to be the highest predictor, which corresponds to the earlier regression findings. If only household income is considered, the group of customers with income between $57,743 and $64,893, with a 53.9 percent response rate, do not appear to be as good a target as those with higher incomes. But IBM SPSS Decision Trees can go beyond simple linear regression to explore further interactions between customer characteristics, allowing the interactions between predictors to define themselves, deriving right from the data instead of having to be defined by the analyst.
When the details of the next level of branches are also used to compare segments, we find that households with income of between $57,743 and $64,893 who also purchased from product class “AB” (Node 8 in Chart 6 and Chart 7) are 21.8 percent more likely to respond to Offer 1 than households in Node 10, which have a higher household income but purchased from product classes “C2” and “DE”.
[Download PDF to see Chart 7]
IBM SPSS Decision Trees gives us a much clearer picture of the subsegments and the set of criteria which truly make up our “best customers” than earlier types of analysis did. We will be able to use this more detailed view to more accurately forecast sales and improve our marketing efforts.
[Download PDF to see Table 12]
Taking action
Through the analyses described here, IBM SPSS Statistics enabled us to quickly analyze our data so that we could learn some important things about our typical customers. We learned that they tend to be longer-term customers from suburban areas. They also are likely to have higher-than-average incomes, and have not responded well, on the whole, to Offer 3.
In addition, by using powerful predictive modeling and segmentation techniques to identify relationships, we developed a model that describes the relationship between income and total money spent to help predict future sales. We also identified unique customer segments by their likelihood to respond to Offer 1.
By comparing multiple characteristics and groups, IBM SPSS Statistics helped us learn more about underlying patterns. Not only was Offer 3 the least lucrative for us, it was particularly unproductive in urban areas, which tended to respond less enthusiastically to our offers than the other two areas did. The fact that customers in urban areas had the lowest average income helps explain their relatively low response to our offers. By identifying such groups of customers, we can better target marketing and customer retention programs.
For instance, because higher-income households show greater revenue potential, we might offer them additional products and services, or develop customer retention programs that help keep them as satisfied, long-term customers. Alternatively, we might find that while customers in urban areas did not in general respond well to our offers, women of a particular income level in that area did, suggesting that it might be appropriate to target them in a certain type of campaign.
As a result of the analyses we conducted, we might make the following plans:
- Build a new customer retention program for our best customers, those defined as higher-income, long-time customers in the suburban area who purchase from product class “AB”.
- Develop and test a new bundle of products and services to better target the needs of the lower-income urban area customers and prospects.
- Repeat sales development of the rural area in the urban and suburban areas to build long-time customers.
- Duplicate Offer 1 to prospects in rural areas.
- Match the funds of future marketing campaigns to the predicted segment profitability (based initially on household income).
Conclusion
This paper describes just a few of the ways that you can use predictive analytics to better understand your customers. By seeing your customers from a number of different perspectives, you can plan more effective programs and systematically measure results. In this way, you’ll build stronger relationships with the customers you value most and decrease the costs of serving less valuable customer segments.
Other IBM SPSS products enable you to anticipate change in your customers’ preferences and behavior. Predictive analytic solutions enable you to proactively plan your business strategies and provide a strong competitive advantage in any industry.
For the purposes of this paper, however, we have shown that the IBM SPSS Statistics product family provides a wide range of analytic options, available in a single, integrated product suite. Even if you’re not a statistician, you can apply this information to market more effectively, retain your most valuable customers, and increase the profitability of your business.
About IBM Business Analytics
IBM Business Analytics software delivers complete, consistent and accurate information that decision-makers trust to improve business performance. A comprehensive portfolio of business intelligence, predictive analytics, financial performance and strategy management, and analytic applications provides clear, immediate and actionable insights into current performance and the ability to predict future outcomes. Combined with rich industry solutions, proven practices and professional services, organizations of every size can drive the highest productivity, confidently automate decisions and deliver better results.
As part of this portfolio, IBM SPSS Predictive Analytics software helps organizations predict future events and proactively act upon that insight to drive better business outcomes. Commercial, government and academic customers worldwide rely on IBM SPSS technology as a competitive advantage in attracting, retaining and growing customers, while reducing fraud and mitigating risk. By incorporating IBM SPSS software into their daily operations, organizations become predictive enterprises – able to direct and automate decisions to meet business goals and achieve measurable competitive advantage. For further information or to reach a representative visit www.ibm.com/spss.
Want more like this?
Want more like this?
Insight delivered to your inbox
Keep up to date with our free email. Hand picked whitepapers and posts from our blog, as well as exclusive videos and webinar invitations keep our Users one step ahead.
By clicking 'SIGN UP', you agree to our Terms of Use and Privacy Policy
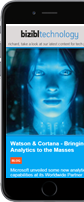
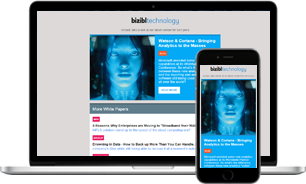
By clicking 'SIGN UP', you agree to our Terms of Use and Privacy Policy